Overview
Machine learning (ML) systems are achieving remarkable performances at the cost of increased complexity. Hence, they become less interpretable, which may cause distrust. As these systems are pervasively being introduced to critical domains, such as medical image computing and computer assisted intervention (MICCAI), it becomes imperative to develop methodologies to explain their predictions. Such methodologies would help physicians to decide whether they should follow/trust a prediction or not. Additionally, it could facilitate the deployment of such systems, from a legal perspective. Ultimately, interpretability is closely related with AI safety in healthcare.
However, there is very limited work regarding interpretability of ML systems among the MICCAI research. Besides increasing trust and acceptance by physicians, interpretability of ML systems can be helpful during method development. For instance, by inspecting if the model is learning aspects coherent with domain knowledge, or by studying failures. Also, it may help revealing biases in the training data, or identifying the most relevant data (e.g., specific MRI sequences in multi-sequence acquisitions). This is critical since the rise of chronic conditions has led to a continuous growth in usage of medical imaging, while at the same time reimbursements have been declining. Hence, improved productivity through the development of more efficient acquisition protocols is urgently needed.
This workshop aims at introducing the challenges & opportunities related to the topic of interpretability of ML systems in the context of MICCAI.
Scope
Interpretability can be defined as an explanation of the machine learning system. It can be broadly defined as global, or local. The former explains the model and how it learned, while the latter is concerned with explaining individual predictions. Visualization is often useful for assisting the process of model interpretation. The model’s uncertainty may be seen as a proxy for interpreting it, by identifying difficult instances. Still, although we can find some approaches for tackling machine learning interpretability, there is a lack of formal and clear definition and taxonomy, as well as general approaches. Additionally, interpretability results often rely on comparing explanations with domain knowledge. Hence, there is need for defining objective, quantitative, and systematic evaluation methodologies.
Covered topics include but are not limited to:
- Definition of interpretability in context of medical image analysis.
- Visualization techniques useful for model interpretation in medical image analysis.
- Local explanations for model interpretability in medical image analysis.
- Methods to improve transparency of machine learning models commonly used in medical image analysis.
- Textual explanations of model decisions in medical image analysis.
- Uncertainty quantification in context of model interpretability.
- Quantification and measurement of interpretability.
- Legal and regulatory aspects of model interpretability in medicine.
Program
The program of the workshop includes keynote presentations of experts working in the field of interpretability of machine learning. A selection of submitted manuscripts will be chosen for short oral presentations (10 minutes + 3 minutes Q&A) alongside the keynotes. Finally, we will have a group discussion which leaves room for a brainstorming on the most pressing issues in interpretability of machine intelligence in the context of MICCAI.
Keynote speaker - 12:30-13:15
Accepted Contributions - 13:15-14:00
Coffee break - 14:00-14:30
Keynote speaker - 14:30-15:15
Accepted Contributions - 15:15-16:15
Keynote speakers
-
Alex Binder, Singapore University of Technology and Design, Sinpagore.
Title: Resolving challenges in deep learning-based analyses of histopathological images using explanation methods
-
Bolei Zhou, Chinese University of Hong Kong.
Title: Interpreting Latent Semantics in GANs for Semantic Image Editing
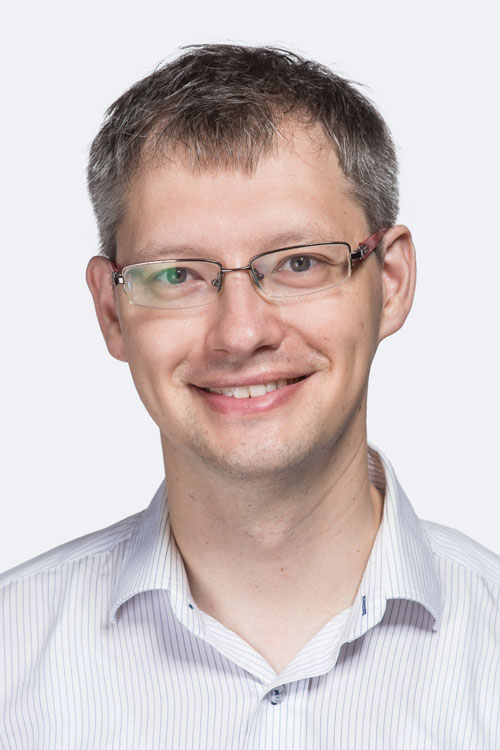
Abstract: Deep learning has recently gained popularity in computational medicine due to its high prediction potential. Recently, explanation methods have emerged, which are so far still rarely used in medicine. Firstly, we explore recent advances in semi-automatic discovery of prediction strategies and biases, demonstrated by applying explanation methods on reinforcement learning tasks. Secondly, we show their application to generate heatmaps that allow to resolve common challenges encountered in deep learning-based digital histopathology analyses. We study binary classification tasks of tumor tissue discrimination in publicly available haematoxylin and eosin slides of various tumor entities and investigate three types of biases: (1) biases which affect the entire dataset, (2) biases which are by chance correlated with class labels and (3) sampling biases. While standard analyses focus on sample-level evaluation, we advocate pixel-wise heatmaps, which are shown to not only detect but also to be helpful to remove the effects of common hidden biases, improving generalization within and across datasets. Explanation techniques are thus demonstrated to be a helpful and highly relevant tool for the development and the deployment phases within the life cycle of real-world applications in digital pathology.
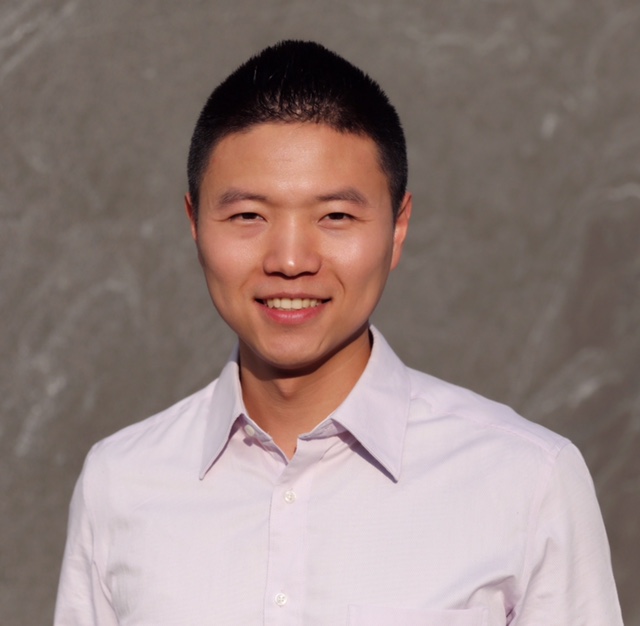
Abstract: Recent progress in deep generative models such as Generative Adversarial Networks (GANs) and Variational Autoencoders (VAEs) has enabled synthesizing photo-realistic images, such as faces and scenes. There have been a lot of interpretability work on visualizing and interpreting the deep representations learned from classifying images, however, it remains much less explored on what has been learned inside the deep representations learned from synthesizing images. In this talk, I will present some of our recent progress in discovering the latent semantics hidden inside the GANs. Identifying these semantics not only allows us to better understand the internal mechanism in generative models, but also facilitates high-fidelity semantic photo editing applications.
Paper submission
Authors should prepare a manuscript of 8 pages, including references. The manuscript should be formatted according to the Lecture Notes in Computer Science (LNCS) style. All submissions will be reviewed by 3 reviewers. The reviewing process will be single-blinded. Authors will be asked to disclose possible conflict of interests, such as cooperation in the previous two years. Moreover, care will be taken to avoid reviewers from the same institution as the authors. The selection of the papers will be based on their relevance for medical image analysis, significance of results, technical and experimental merit, and clear presentation.
We intend to join the MICCAI Satellite Events joint proceedings, and publish the accepted papers as LNCS. We are also considering making the pre-print of the accepted papers publicly available.
Click here to submit your paper.
Important dates
- Opening of submission system (Mid June).
- Submission deadline (July 18th).
- Reviews due (August 5th).
- Notification of acceptance (August 12th).
- Camera-ready papers (August 19th).
Organizers
- Mauricio Reyes, University of Bern, Switzerland.
- Ender Konukoglu, ETHZ, Switzerland.
- Ben Glocker, Imperial College, U.K
- Roland Wiest, University Hospital, Bern
Programm Committee
- Bjoern Menze, Technical University of Munich, Germany.
- Carlos A. Silva, University of Minho, Portugal.
- Dwarikanath Mahapatra, IBM Research, Australia.
- Nick Pawlowski, Imperial college London, U.K.
- Hrvoje Bogunovic, Medical University of Vienna, Austria.
- Wilson Silva,University of Porto, Portugal
- Islem Rekik, Istanbul Technical University, Turkey.
- Raphael Meier, University Hospital Bern, Switzerland.
- Sérgio Pereira, University of Minho, Portugal.