This workshop is part of the MICCAI 2020 conference.
The workshop will be 100% remote.
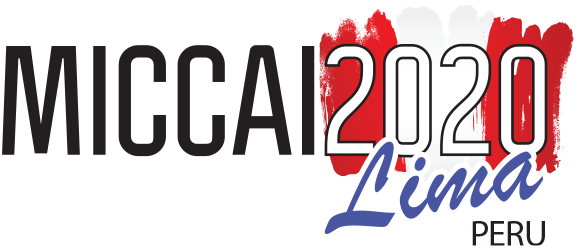
Overview
Machine learning (ML) systems are achieving remarkable performances at the cost of increased complexity. Hence, they become less interpretable, which may cause distrust. As these systems are pervasively being introduced to critical domains, such as medical image computing and computer assisted intervention (MICCAI), it becomes imperative to develop methodologies to explain their predictions. Such methodologies would help physicians to decide whether they should follow/trust a prediction or not. Additionally, it could facilitate the deployment of such systems, from a legal perspective. Ultimately, interpretability is closely related with AI safety in healthcare.
However, there is very limited work regarding interpretability of ML systems among the MICCAI research. Besides increasing trust and acceptance by physicians, interpretability of ML systems can be helpful during method development. For instance, by inspecting if the model is learning aspects coherent with domain knowledge, or by studying failures. Also, it may help revealing biases in the training data, or identifying the most relevant data (e.g., specific MRI sequences in multi-sequence acquisitions). This is critical since the rise of chronic conditions has led to a continuous growth in usage of medical imaging, while at the same time reimbursements have been declining. Hence, improved productivity through the development of more efficient acquisition protocols is urgently needed.
The Workshop on Interpretability of Machine Intelligence in Medical Image Computing (iMIMIC) at MICCAI 2020 aims at introducing the challenges & opportunities related to the topic of interpretability of ML systems in the context of MICCAI.
Scope
Interpretability can be defined as an explanation of the machine learning system. It can be broadly defined as global, or local. The former explains the model and how it learned, while the latter is concerned with explaining individual predictions. Visualization is often useful for assisting the process of model interpretation. The model’s uncertainty may be seen as a proxy for interpreting it, by identifying difficult instances. Still, although we can find some approaches for tackling machine learning interpretability, there is a lack of formal and clear definition and taxonomy, as well as general approaches. Additionally, interpretability results often rely on comparing explanations with domain knowledge. Hence, there is the need for defining objective, quantitative, and systematic evaluation methodologies.
Covered topics include but are not limited to:
- Definition of interpretability in context of medical image analysis.
- Visualization techniques useful for model interpretation in medical image analysis.
- Local explanations for model interpretability in medical image analysis.
- Methods to improve transparency of machine learning models commonly used in medical image analysis.
- Textual explanations of model decisions in medical image analysis.
- Uncertainty quantification in context of model interpretability.
- Quantification and measurement of interpretability.
- Legal and regulatory aspects of model interpretability in medicine.
Program
The program of the workshop includes keynote presentations of experts working in the field of interpretability of machine learning. A selection of submitted manuscripts will be chosen for short oral presentations (10 minutes + 3 minutes Q&A) alongside the keynotes. Finally, we will have a group discussion which leaves room for a brainstorming on the most pressing issues in interpretability of machine intelligence in the context of MICCAI.
Final program:
UTC time - October 4th, 2020
Keynote speakers
-
Himabindu Lakkaraju, Harvard University, USA.
Title: Understanding the Limits of Explainability in ML-Assisted Decision Making
Abstract: As machine learning black boxes are increasingly being deployed in domains such as healthcare and criminal justice, there is growing emphasis on building tools and techniques for explaining these black boxes in an interpretable manner. Such explanations are being leveraged by domain experts to diagnose systematic errors and underlying biases of black boxes. In this talk, I will demonstrate that post hoc explanations techniques that rely on input perturbations, such as LIME and SHAP, are not reliable. Specifically, I will discuss a novel scaffolding technique that effectively hides the biases of any given classifier by allowing an adversarial entity to craft an arbitrary desired explanation. Our approach can be used to scaffold any biased classifier in such a way that its predictions on the input data distribution still remain biased, but the post hoc explanations of the scaffolded classifier look innocuous. Using results from real world datasets (including COMPAS), I will demonstrate how extremely biased (racist) classifiers crafted by our framework can easily fool popular explanation techniques such as LIME and SHAP into generating innocuous explanations which do not reflect the underlying biases. I will conclude the talk by discussing extensive user studies that we carried out with domain experts in law to understand the perils of such misleading explanations and how they can be used to manipulate user trust.
-
Wojciech Samek, Fraunhofer HHI, Germany.
Title: Extending Explainable AI Beyond Deep Classifiers
Abstract: Over the years, ML models have steadily grown in complexity, gaining predictivity often at the expense of interpretability. Explainable AI (or XAI) has emerged with the goal to produce models that are both predictive and understandable. XAI has reached important successes, such as robust heatmap-based explanations of DNN classifiers. From an application perspective, there is now a need to massively engage into new scenarios such as explaining unsupervised / reinforcement learning and non-neural network type models ML models, as well as producing explanations that are optimally structured for the human, and explanations. This talk will summarize recent development in extending XAI beyond deep classifiers.
Best paper award
Winner of a pecuniary award of 300€ for the best paper:
Projective Latent Interventions for Understanding and Fine-tuning Classifiers by Andreas Hinterreiter, Marc Streit, Bernhard Kainz.
Paper submission
Authors should prepare a manuscript of 8 pages, excluding references. The manuscript should be formatted according to the Lecture Notes in Computer Science (LNCS) style. All submissions will be reviewed by 3 reviewers. The reviewing process will be single-blinded. Authors will be asked to disclose possible conflict of interests, such as cooperation in the previous two years. Moreover, care will be taken to avoid reviewers from the same institution as the authors. The selection of the papers will be based on their relevance for medical image analysis, significance of results, technical and experimental merit, and clear presentation.
We intend to join the MICCAI Satellite Events joint proceedings, and publish the accepted papers as LNCS. We are also considering making the pre-print of the accepted papers publicly available.
There is also a Special Issue of the workshop, published by the Machine Learning and Knowledge Extraction journal, which is open to outside contributions.
Click here to submit your paper.
Important dates
- Opening of submission system: Mid May 2020.
- Paper Submission due:
June 30, 2020.July 14, 2020. - Notification of paper decisions:
July 21, 2020.August 5, 2020. - Camera-ready papers due:
July 31, 2020.August 15, 2020. - Workshop: 4 October 2020, 9:00-13:00 (UTC time)
Venue
The iMIMIC workshop will be held in the morning of 4 of October as a workshop of MICCAI 2020.
We would like to inform you that in light of the ongoing COVID-19 pandemic, the MICCAI 2020 Conference Organizing team and the MICCAI Society Board have decided to hold the MICCAI 2020 annual meeting planned for October 4-8, 2020 in Lima, Peru as a fully virtual conference.
More information regarding the venue can be found at the conference website
Committees
General Chairs
- Jaime S. Cardoso, INESC TEC and University of Porto, Portugal.
- Pedro H. Abreu, CISUC and University of Coimbra, Portugal.
- Ivana Isgum, Amsterdam University Medical Center, The Netherlands
Publicity Chair
- Jose P. Amorim, CISUC and University of Coimbra, Portugal.
Program Chair
- Wilson Silva, INESC TEC and University of Porto, Portugal.
Sponsor Chair
- Ricardo Cruz, INESC TEC and University of Porto, Portugal.
Program Committee
- Ben Glocker, Imperial College, United Kingdom.
- Bettina Finzel, University of Bamberg, Germany.
- Carlos A. Silva, University of Minho, Portugal.
- Christoph Molnar, Ludwig Maximilian University of Munich, Germany.
- Claes Nøhr Ladefoged, Rigshospitalet, Denmark.
- Dwarikanath Mahapatra, Inception Institute of AI, Abu Dhabi, UAE.
- George Panoutsos, University of Sheffield, United Kingdom.
- Hrvoje Bogunovic, Medical University of Vienna, Austria.
- Isabel Rio-Torto, University of Porto, Portugal.
- Joana Cristo Santos, University of Coimbra, Portugal.
- Kelwin Fernandes, NILG.AI, Portugal.
- Luis Teixeira, University of Porto, Portugal.
- Miriam Santos, University of Coimbra, Portugal.
- Nick Pawlowski, Imperial College London, United Kingdom.
- Ricardo Cruz, INESC TEC and University of Porto, Portugal.
- Sérgio Pereira, Lunit, South Korea.
Sponsors
We thank our sponsors.
Interested in participating and being a sponsor? Email us: ricardo.pdm.cruz@inesctec.pt